Acoustic signal enhancement using autoregressive PixelCNN architecture
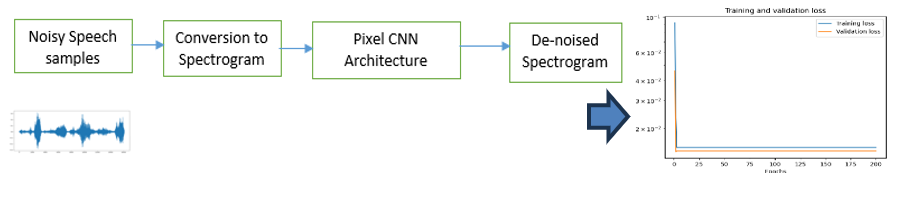
DOI:
https://doi.org/10.62110/sciencein.jist.2024.v12.770Keywords:
Pixel CNN, deep generative model, auto regression, non-stationary noises, speech de-noisingAbstract
Acoustic Signals such as speech and sound are easily degraded by interferences present in our surroundings.The present work explores the usage of the Pixel CNN architecture for the removal of non-stationary noises from the speech signal. The presence of noise in speech signals affects the performances of applications that use speech signal as a medium for communication such as automatic speech recognition systems, hearing aid, mobile phones. Pixel CNN is a deep generative network architecture implemented as an autoregressive model. The dataset “NOIZEUS” is used for noise mixed speech samples and clean speech samples. The architecture learns the feature from the input speech using the spectrogram representation of speech signal. To prove the efficiency of the method, the performance of Pixel CNN architecture is compared with a number of baseline methods to prove its efficiency. The parameters used for comparison are “PESQ” and “STOI”.
URN:NBN:sciencein.jist.2024.v12.770
Downloads
Downloads
Published
Issue
Section
URN
License
Copyright (c) 2023 Shibani Kar

This work is licensed under a Creative Commons Attribution-NonCommercial-NoDerivatives 4.0 International License.
Rights and Permission