A deep learning approach for underwater fish detection
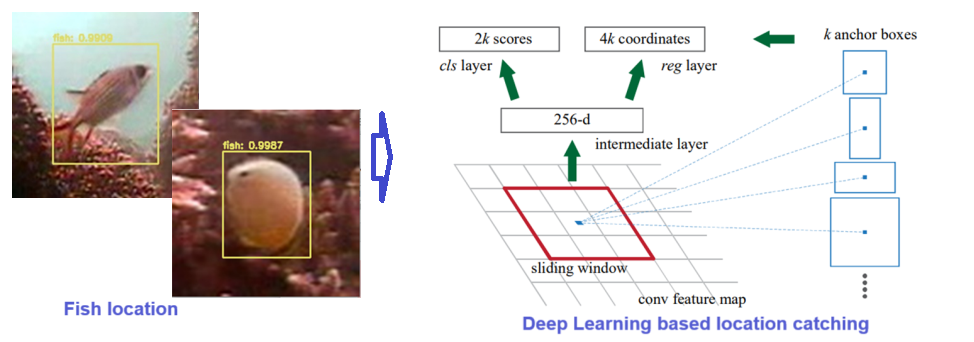
DOI:
https://doi.org/10.62110/sciencein.jist.2024.v12.765Keywords:
Deep learning, anchor box, Object Detection, underwater images, YOLOv3, Faster R-CNN, MobileNetAbstract
Identifying moving objects in video sequences is crucial for various applications, including underwater surveillance, biomedical detection, threat identification, defense, and navy. Oceanic environments often have deteriorating images due to water medium properties. In static conditions, the background remains stationary, while in dynamic conditions, both the background and foreground exhibit motion, making it difficult to differentiate between them. Automation of detection systems is essential for these applications. Therefore, the suggested system offers a deep learning-based solution for underwater fish recognition that employs three models: YOLO V3, SSD Mobile net V2, and Faster RCNN Resnet 50, all of which were trained on a bespoke dataset called Fish4knowledge. The algorithms have been taught to recognize and reliably pinpoint fish species in underwater photos and videos. To improve performance, data pre-treatment, model selection, and hyperparameter adjustment are carried out. The best-performing model is chosen after evaluation on a different validation dataset. The proposed method provides a viable solution for real-time underwater fish detection, which will aid in marine biology research, environmental monitoring, and autonomous underwater vehicles (AUVs). Model updates and adaption to changing undersea conditions are required for long-term accuracy and performance enhancement. The research results demonstrate that the SSD Mobilenet v2 model gives the highest precision value of 98.21% as compared to the YOLO V3 and Faster RCNN Resnet50 models.
URN:NBN:sciencein.jist.2024.v12.765
Downloads
Downloads
Published
Issue
Section
URN
License
Copyright (c) 2023 Vrushali Pagire, Anuradha Phadke, J Hemant

This work is licensed under a Creative Commons Attribution-NonCommercial-NoDerivatives 4.0 International License.
Rights and Permission