RRBCNN: Aircraft detection and classification using Bounding Box Regressor based on Scale Reduction module
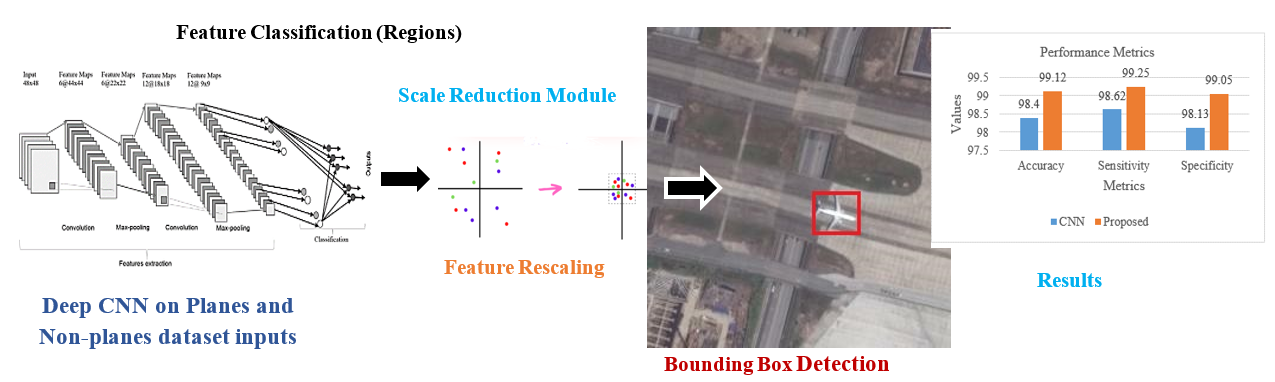
Abstract
Research on aircraft detection has recently resulted in significant military and civil applications. Concurrently, research relying on DL (Deep Learning) and image processing has gained wide interest in object tracking. However, variations in the kind of aircraft, complex background, and pose have made it complex to detect aircraft efficiently. Thus, an effective algorithm is needed to solve this difficulty. For this purpose, the study considers the plane-sent dataset. It proposes RRBCNN (Region Regression Based Convolutional Neural Network) for classifying stationary aircraft into "planes" and "not planes" with high accuracy. The study also introduces SRM (Scale Reduction Module), which RRBCNN utilizes for minimizing the feature-map scaling. It also eliminates information loss to enhance the training rate. This assists in improving aircraft detection by affording suitable bounding boxes. The performance of the proposed system is analyzed in terms of AC (Accuracy), FPR (False Positive Rate), MR (Missing Rate), ER (Error Ratio), precision, average processing time (T), recall, specificity, sensitivity, and FN (False Negative) rate. Three conventional types of research are considered for comparative analysis to evaluate the efficiency of the proposed work. Finally, the efficiency of the introduced methodology is proved through analysis.
URN:NBN:sciencein.jist.2023.v11.412
Downloads
Downloads
Published
Issue
Section
URN
License
Copyright (c) 2022 Bhavani Sankar Panda, Kakita Murali Gopal, Rabi Narayan Satapathy

This work is licensed under a Creative Commons Attribution-NonCommercial-NoDerivatives 4.0 International License.
Rights and Permission